ATM Transaction Status Feature Analysis and Anomaly Detection
Abstract
In this paper, based on ATM transaction status analysis and anomaly detection problem, by analyzing the transaction statistics of a bank ATM application system, the characteristic parameters of ATM transaction status are extracted and analyzed, then a set of targets are designed. An abnormal monitoring scheme that can promptly and accurately alarm the four abnormal situations in which the trading volume is steep, the transaction failure rate is increased, the transaction processing is slow, and the transaction response time is too long. Firstly, the transaction data is divided to distinguish between working days, non-working days, trading volume troughs, and normal trading periods, to avoid data interference between different time periods and to take into account the data discontinuity. The characteristics of the anomaly data were identified by K-Means Clustering Analysis. Then the data is analyzed by B-P Neural Network method, the change rule of ATM transaction status with time is obtained. According to this rule, the ATM transaction status is judged, and the abnormal situation is alarmed in time. Finally, this paper increases the amount and type of data collected, then increases the influencing factors such as ATM popularity, holidays, transaction types into the model, uses the existing transaction data before and after the Spring Festival to verify, in order to obtain a more realistic monitoring and early warning program. The transaction status anomaly monitoring scheme designed in this paper not only can correctly judge but also timely alarm the financial aid equipment failure scenarios, so that the security of the financial self-service equipment trading system is guaranteed.
Full Text:
PDFDOI: https://doi.org/10.11114/set.v6i1.3829
Refbacks
- There are currently no refbacks.
Studies in Engineering and Technology ISSN 2330-2038 (Print) ISSN 2330-2046 (Online)
Copyright © Redfame Publishing Inc.
To make sure that you can receive messages from us, please add the 'redfame.com' domain to your e-mail 'safe list'. If you do not receive e-mail in your 'inbox', check your 'bulk mail' or 'junk mail' folders.
If you have any questions, please contact: set@redfame.com
-------------------------------------------------------------------------------------------------------------------------------------------------------------
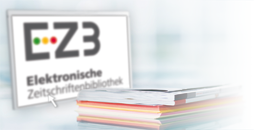